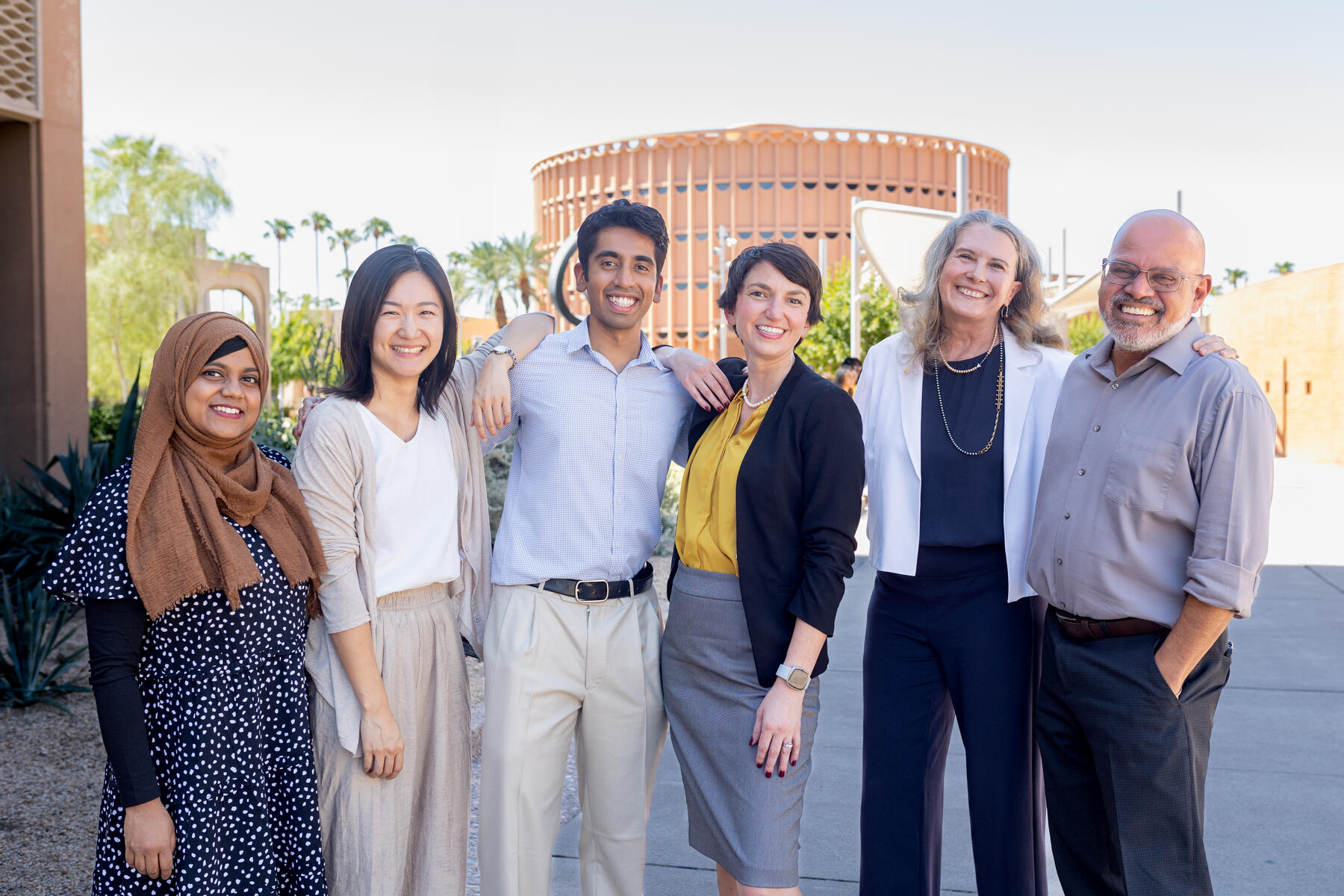
Evaluation framework sets a new benchmark for ethical AI
The upside of generative artificial intelligence (AI) is tremendous. For example, large language models (LLMs) can generate text for reports, outlines or articles, automate repetitive tasks and quickly synthesize immense amounts of data. Other AI tools can create stunning visuals, tailored audio and enhance human creativity.
Yet the AI Acceleration team in Arizona State University’s (ASU) Enterprise Technology department is safeguarding against AI’s potential downsides – namely biases related to race, gender and class that can arise from how custom GPTs are trained. As such, they have recently released an evaluation framework to set a new standard for the use of ethical AI.
The HigherEd Language Model Evaluation Framework is a technical paper for a technical audience. The 10-page document details a comprehensive approach to evaluating LLMs and AI-powered chatbots in the context of higher education. Designed for both external vendor solutions and internally developed AI applications, it ensures these tools align with the values and adhere to the ethical innovation principles of academic institutions.
The framework has two components: a human evaluation process and an automated evaluation.
The automated process is developed through code and is called the Ethical AI Engine. The tool will soon be a feature on ASU’s Create AI and MyAI Builder platforms, and once it is integrated, all chatbots at ASU will have to run through the test.
(Stella) Wenxing Liu is the lead data scientist on the AI Acceleration team and was integral in designing this portion of the framework.
“We used the Ethical AI Engine to run tests on vendor solutions,” she said. “It’s very straightforward to determine which one is significantly better than the other.”
Liu added that the test is more nuanced than a pass-or-fail system. Instead, the engine generates a score between zero and one for different dimensions, which include accuracy, robustness, fairness, bias and efficiency. These scores are then compared to standard base model GPTs. Currently, all 20 LLMs in MyAI Builder have scored up to standard, according to the Ethical AI Engine evaluation.
“We will have explanations under each of the metrics. We want to show problematic metrics in red to indicate this might be something worth looking into,” Liu said.
For a chatbot to be adopted for use at ASU, passing the automated test is not enough. Liu considers the Ethical AI Engine as a “preliminary screening,” and said the human evaluation component, while more theoretical and less tangible, provides a true comprehensive assessment.
“The human evaluation part is a design, it’s a framework, it’s essentially a workflow that we follow,” she said, adding that the purpose of the human evaluation is to help assess usability and real-life effectiveness.
With an eye toward the future, the AI Acceleration team will eventually integrate more dimensions to their framework and add a third layer to the evaluation process – a mechanism to monitor chatbots and how they evolve. This ensures that even after passing a rigorous initial vetting, the chatbot will continue to adhere to the responsible innovation standards of ASU.
While Liu’s team has been using this framework to evaluate chatbots regularly, they aim to release the Ethical AI Engine on the Create AI platform in October. This will allow AI bot builders to understand how their GPT performs early in the design process.
With the goal of evaluating for bias, accuracy, integrity and fairness, the AI Acceleration team views the framework as a way to establish benchmarks and shows ASU's commitment to creating AI that serves humanity.