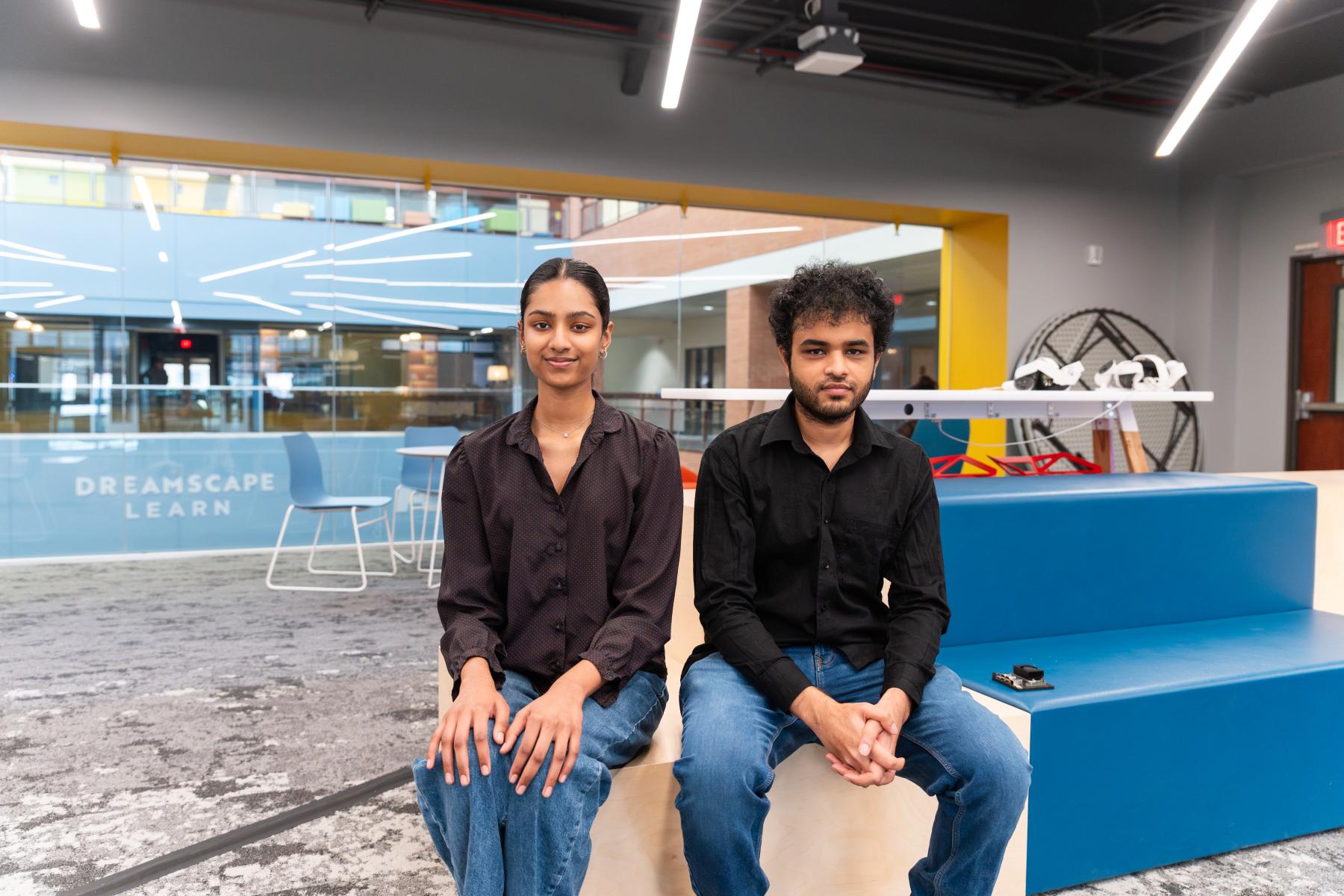
Students at Next Lab work to bring AI to all
This story was first published as part of the spring 2025 edition of The AI Journey at ASU.
As part of a broader effort to bridge digital divides and connect underserved communities with cutting-edge resources, a team of student workers at ASU Next Lab is working to develop a transformative solution: EDge AI. The initiative’s name highlights the concept of education at "the edge" — reaching communities where access to technology, especially AI, is limited due to financial barriers, lack of internet access, or even unreliable power.
Ananya Bhargavi Kodali, Hrushikesh Pandit, Hithesh Rai Purushothama and Sahil Garg are student workers at ASU Next Lab, working with Dan Munnerley, executive director.
“Our main motivation was around equity and accessibility for who can use these resources,” said Ananya Bhargavi Kodali.
AI is typically a paid-for service delivered over the internet, demanding considerable resources in terms of power and consumption. These prohibitive requirements often impede communities who stand to benefit the most from access to robust digital tools.
The team at Next Lab strives to dismantle these obstacles with EDge AI, which is designed with optimization and accessibility in mind.
EDge AI functions without an internet connection and on various devices, using minimal power, all while boasting a user-friendly interface. Since EDge AI can be run locally, users enjoy the added advantage of privacy and security. This offers meaningful benefits to indigenous groups, for example, who endeavor to protect their cultural heritage by carefully supervising the tools used to distribute or transform their intellectual property.
EDge AI has a bold vision to deliver quality AI experiences despite structural and environmental limitations. The student team has been running EDge AI prototypes on different devices, the smallest of which are Raspberry Pis — single-board computers that can be held in the palm of one’s hand. The large language model — Llama from Meta — along with the database to act as the knowledge source are uploaded to the devices to offer the offline AI experience.
“By running a [large language] model on the device and training it on the database, you can create a chatbot that works offline,” said Hrushikesh Pandit.